The primary objective of synthetic biology is to conceptualize, engineer, and construct novel biological components, devices, and systems based on established principles and extant information or to reconfigure existing natural biological systems. The core concept of synthetic biology encompasses the design, modification, reconstruction, or fabrication of biological components, reaction systems, metabolic pathways and processes, and even the creation of cells and organisms with functions or living characteristics. This burgeoning field offers innovative technologies to address challenges with sustainable development in environment, resource, energy, and so on. Undeniably, synthetic biology has yielded significant progress in numerous fields, ranging from DNA recombination to gene circuit design, yet its full potential remains insufficiently explored, but the emergence and application of artificial intelligence (AI) definitely can facilitate the development of synthetic biology for more applications. From a synthetic biology perspective, essence for life is rooted in digitalization and designability. This article reviews current advances in computational biology, particularly AI for synthetic biology to be more efficient and effective, focusing on the development of biocatalysts, regulators, and sensors. De novo enzyme design has been successfully implemented by using Rosetta software, as AI exhibiting significant potential for generating innovative structures and protein sequences with diverse functions. Also, the reprogramming of natural enzymes for specific purposes is crucial for synthetic biology applications. By employing various force fields and sampling techniques, promiscuity and thermal stability can be modified to accommodate specific requirements rather than those with natural hosts. AI can be integrated into the life-cycle of synthetic biology through an active learning paradigm, which enables alterations in enzyme specificity, and demonstrates potential for accurately and rapidly predicting mutation effects, surpassing force-field-based methods. The rapidly decreasing cost of sequencing has facilitated the characterization of cis-regulators, primarily DNA and RNA, with high-throughput. Concurrently, more trans-regulators have been identified in sequenced genomes. The expanding wealth in big data serves as a driving force for AI. AI models have successfully predicted the strength of promoters, ribosome binding sites (RBSs), and enhancers, and generated artificial protomers and RBSs. Recent progress in RNA structure prediction is expected to aid the design of RNA elements. Sensors, vital for genetic circuits and other applications such as toxin detection, typically involve interactions among various molecules, including nucleic acids, proteins, small organic molecules, and metal ions. Consequently, sensor design necessitates the integration of diverse computational biology tools to balance accuracy and computational cost. As the pool of data keeps growing, we anticipate that AI will be increasingly applied to the design of more bio-parts.
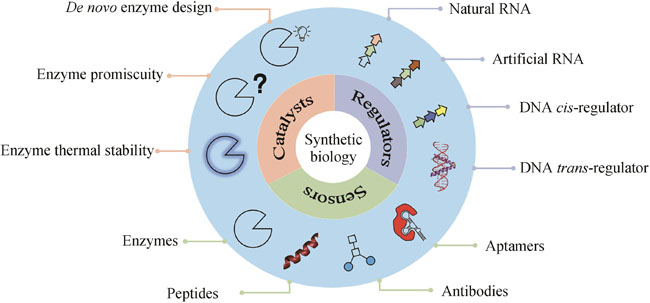