Protein engineering is one of the important research fields of synthetic biology. However, de novo design of protein functions based on rational design is still challenging, because of the limited understanding on biological fundamentals such as protein folding and the natural evolution mechanism of enzymes. Directed evolution is capable of optimizing protein functions effectively by mimicking the principle of natural evolution in the laboratory without relying on structure and mechanism information. However, directed evolution is highly dependent on high-throughput screening methods, which also limits its applications on proteins which lack high-throughput screening methods. In recent years, artificial intelligence has been developed very rapidly for integrating into multidisciplinary fields. In synthetic biology, artificial intelligence-assisted protein engineering has become an efficient strategy for protein engineering besides rational design and directed evolution, which has shown unique advantages in predicting the structure, function, solubility of proteins and enzymes. Artificial intelligence models can learn the internal properties and relationships from given sequence-function data sets to make predictions on properties for virtual sequences. In this article, we review the application of artificial intelligence-assisted protein engineering. With the basic and process of the strategy introduced, three key points that affect the performance of the predictive model are analyzed: data, molecular descriptors and artificial intelligence algorithms. In order to provide useful tools for researchers who want to take advantage of this strategy, we summarize the main public database, diverse toolkits and web servers of the common molecular descriptors and artificial intelligence algorithms. We also comment on the functions, applications and websites of several artificial intelligence-assisted protein engineering platforms, through which a complete prediction task including protein sequences representation, feature analysis, model construction and output can be completed easily. Finally, we analyze some challenges that need to be solved in the artificial intelligence-assisted protein engineering, such as the lack of high-quality data, deviation in data sets and lacking of the universal models. However, with the development of automated gene annotations, ultra-high-throughput screening technologies and artificial intelligence algorithms, sufficient high-quality data and appropriate algorithms will be developed, which can enhance the performance of artificial intelligence-assisted protein engineering and thus facilitate the development of synthetic biology techniques.
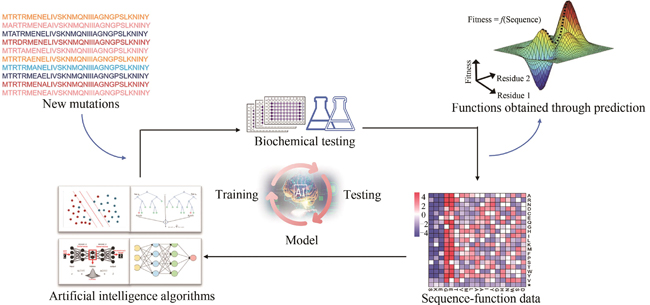